Cameras are used in intelligent transportation systems because they allow acquiring wide range of information about vehicles and situation on the road. Video processing usually is computationally demanding and changing outdoor environment is challenging for computer vision. Here we propose a novel, efficient and adaptive algorithm using intervals on a virtual detection lines for vehicle detection, tracking and classification.
Detection, tracking and classification
Virtual line detectors are placed perpendicular to the road in a video frame. For the computational efficiency only those detection lines are processed. Single line can count passing vehicles. On detection line, intervals are created – passing vehicle is represented by two coordinates on a line. Combining intervals of several lines vehicle objects are created and vehicle parameters can be acquired. Accuracy of detected width, length, height, speed and type of vehicles depends on number of used detection lines. Vehicle movement trough detection lines is also tracked.
Intervals are processed and combined in world plane instead of frame -road and intervals are drawn as they would be seen by observer above the road. Such transform allows to infer interval affiliation to specific vehicles. After calibration of frame scene, transform allows to acquire vehicle parameters in real world units. Spatial parameters of vehicles, detected by algorithm, are used to classify vehicles in to truck and motorcars.
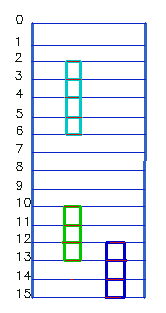
Use of adaptive backgrounds and thresholds allows to detect vehicles and events in different and changing lighting and weather conditions. Automatic detection of night changes detection to night mode. Shutter speed of camera is increased and only headlights of vehicles are detected. Using spatial and temporal information algorithm counts two headlights of same vehicles as single vehicle.
Video processing algorithm is computationally efficient since all processing is carried on a detection lines and not on whole frame. Configuration of number of used detection lines allows convenient compromise between accuracy and available computation power.
Simple detection and counting of vehicles can be accomplished with single detection line which is most limited but also most computationally efficient mode of operation of presented algorithm. Counting with single and multiple lines was tested on videos with different weather and lighting conditions. Test results for vehicle counting with single line showed 94%-99% accuracy for different videos. Best results are obtained by combining intervals from different detection lines in to vehicle objects 95%-100%. Same videos were used to test vehicle classification – approximately 88% of trucks were correctly detected.
Accident detection
Tracking of vehicle
s allows detecting stopped vehicle sand also returns trajectories of vehicles. Stopped vehicles and changes in trajectories of vehicles compared to usual trajectories are used to infer emergency situations on the road. In shown example accident has occurred outside detection region. Also two vehicles have stopped in unusual place on the road. Such situation indicates accident and it may be used as trigger to save last 10 minutes of traffic video. Algorithm has also automatically detected four average trajectories of vehicles in the detection region covered by hidden detection lines and usual probabilities for vehicles to chose those trajectories.
Detection of red light runners
Virtual detection lines can be placed in sections of crossroads which should not be crossed under red light. Multiple lines allow to detect direction of vehicle movement. System may receive input information about current state of traffic light. If it is red and vehicle is crossing the stop line, system detects a violation. Red light runners can be detected also on crosswalks. Vehicles which are moving perpendicularly to detection lines are detected, meanwhile pedestrians move parallel. to virtual lines and are not considered as vehicle objects.
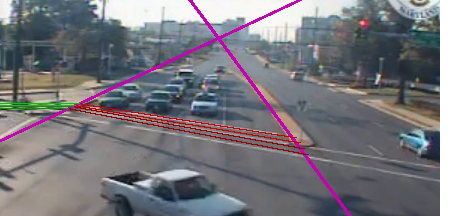
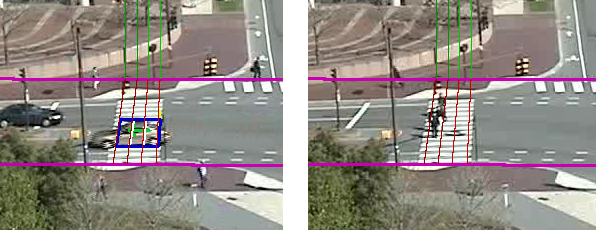
* Details of vehicle detection algorithm are published in: Kadikis R., Freivalds K.: Efficient Video Processing Method for Traffic Monitoring Combining Motion Detection and Background Subtraction. Proceedings of the Fourth International Conference on Signal and Image Processing 2012 (ICSIP 2012) Lecture Notes in Electrical Engineering Volume 211, 2013, pp 131-141.
* Details of vehicle parameter acquisition and classification are described in: Kadikis R., Freivalds K.: Vehicle classification in video using virtual detection lines. Paper has been presented at Sixth International Conference on Machine Vision (ICMV 13).